ImmuneWatch DESIGN
Use machine learning to find epitopes or antigens for your vaccine.

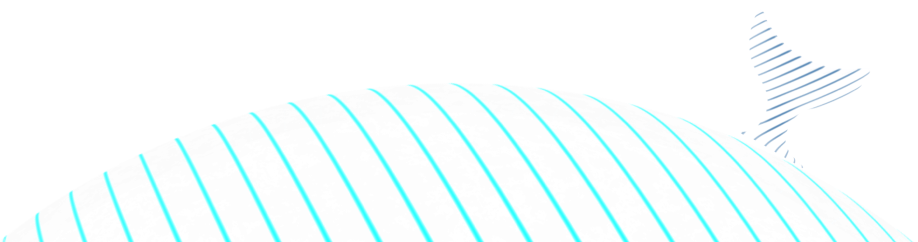
Looking for potent antigens and epitopes to design your vaccine?
Many vaccines have been developed through a trial and error approach, with a large pool of candidates tested in vitro to screen for the most effective ones. This approach can be time-consuming, costly, and not always lead to the most effective vaccines.
Machine learning can address these problems and provide a number of other benefits complementary to traditional vaccine development methods.
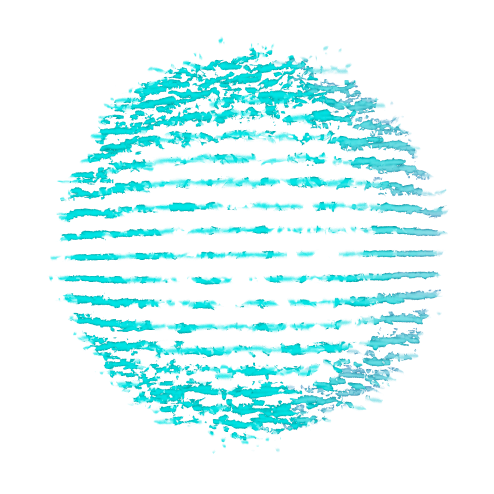

Together with ImmuneWatch we were able to assess vaccine-induced T-cell signatures in our unique longitudinal sample collection of cancer patients. Based on antibody titers, it has previously been shown that cancer patients induce a weaker response towards COVID19 vaccines. T-cell immunity in this context is far less characterized and understood. Thanks to the ImmuneWatch DETECT platform we were able to take a deep dive into the SARS-CoV-2-specific T-cell response of our cancer patient cohort.

ImmuneWatch assisted us in analysing reactive T-cells through TCR sequencing and data anaysis in an oncology setting. Together, we identified potential TCR candidates for further in vitro validation. This collaboration provided us with insights into T-cell reactivity and the immune profile of our stimulation experiments, advancing our understanding and guiding future research.
The DESIGN analysis performed by ImmuneWatch is very informative and useful for making decisions on our different candidate antigens. Thank you for this nice work!

ImmuneWatch DETECT wins Machine Learning competition
We are excited to share our recent win in the IMMREP23 Kaggle competition focused on TCR (T-cell receptor) specificity prediction.
Read the article
Use cases
Our offering
Find the epitope-specificity of your TCRs today
ImmuneWatch DETECT for Academia
Best-in-class TCR-epitope prediction algorithm
Trained on high quality in-house database IMWdb
Executed locally, no data uploaded to the cloud
Explainability of TCR-epitope predictions
Continuously updated to always get the best predictions
10 free credits per month
ImmuneWatch DETECT for Industry
Best-in-class TCR-epitope prediction algorithm
Trained on high quality in-house database IMWdb
Executed locally, no data uploaded to the cloud
Explainability of TCR-epitope predictions
Continuously updated to always get the best predictions
Email support within 3 business days
Try it out on your own data
ImmuneWatch DETECT Service
In-depth data analysis by immunoinformatic experts
Detect pathogen-, vaccine-, therapy-specific TCRs with our best-in-class TCR-epitope prediction algorithm
Possibility to generate data for your epitopes of interest
FAQ
Find more information and answers to frequently asked questions on our documentation pages.
Is ImmuneWatch DETECT Free?
We offer 10 free credits per month for academic users. If you need additional credits—whether you’re from academia or enterprise—you can contact us to purchase more.
What is a credit?
With one credit, you can run ImmuneWatch DETECT on one TCR repertoire sample. You can rerun the same sample multiple times with different settings, and it won’t deduct additional credits.
How long are my credits valid?
Your credits are valid for one year from the time of purchase or allocation.
What is a TCR repertoire sample?
We define a sample based on the type of TCR sequencing data:
• Non-paired data (e.g. Bulk TCR-β data): A single file containing up to 500,000 TCR clonotypes.
• Paired data (e.g. Single Cell TCR-αβ data): A single file containing up to 10,000 TCR clonotypes.
For further questions about purchasing credits or any other inquiries, feel free to contact us.
Can ImmuneWatch DETECT detect any kind of epitope?
ImmuneWatch DETECT is an algorithm that falls into the “seen epitope models” category, where it was found to be the best-in-class. This means that it needs to have training data on an epitope, before it can detect TCRs related to this epitope.
IMWdb currently contains more than 1900 epitopes and is continuously expanded. In addition, we offer a service to generate the data specifically for your epitope of interest. Request a quote here.
A true “unseen epitope model” is currently being researched.
Is ImmuneWatch DETECT only limited to TCR Beta chains?
No, ImmuneWatch DETECT is able to annotate both the Alpha and Beta chains of TCRs. In addition, it can also work Delta and Gamma chains.
Do you also offer TCR sequencing?
Yes, we can help you out with your TCR sequencing project as well. Feel free to contact us for a quote for your project.
Are the results reliable?
A recent public benchmark (IMMREP23) concluded that TCR-epitope prediction models perform very well when they have access to extensive training data on specific (“seen”) epitopes. However, predicting interactions for new (“unseen”) epitopes remains an unsolved issue in the field.
Among the methods tested, ImmuneWatch’s DETECT stood out with what the final manuscript described as a “substantial predictive advantage,” outperforming other models in its category.
Read more about ImmuneWatch DETECT's performance here.
How does your machine learning model work?
ImmuneWatch DETECT is a custom-built probabilistic model that judges if the TCR has enough information to conclude if it is specific to a target epitope. The design choices have been guided by our biological knowledge and years of experience of working with these kind of prediction models.
Even though the model itself is relatively complex, it does offer an intuitive explanation for each prediction by citing the reference TCRs that were used to make the prediction. More info regarding this Explainibility feature can be found on our Documentation pages.
Our offering
Make your vaccine design process more data-driven by implementing machine learning and approaches from reverse vaccinology.
Reverse vaccinology as a service
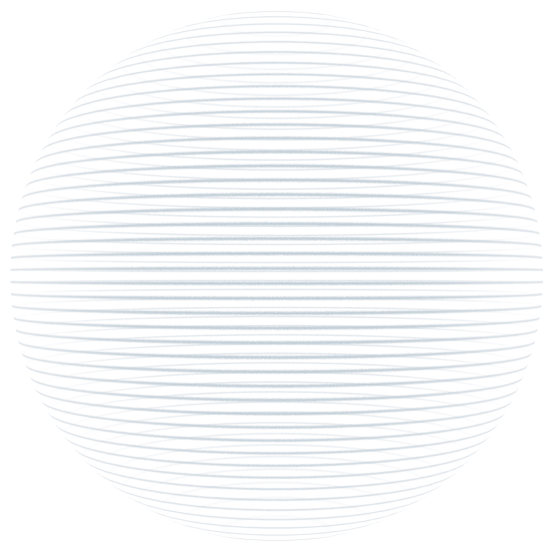