Watch out for negative data bias in machine learning
Machine learning, with its potential to revolutionise the field of immunology, isn’t immune to the pitfalls of biased data. That is a cautious lesson discussed in a new academic publication by ImmuneWatch co-founders Prof. Kris Laukens and Prof. Pieter Meysman.
In this paper, they discuss recent work by Gao et al., who sought to predict TCR–epitope bindings using an unseen epitope model, achieving a reported ROC-AUC of 70.8%. Yet, a new evaluation by the team at the University of Antwerp showed that biases in the negative data used to train the model resulted in performance dropping to random levels when tested in real-world scenarios.
For context, biases in machine learning data aren’t a new phenomenon. An illustrative example is a classifier designed to identify malignant skin lesions, which instead learned to identify rulers in images. Such biases, whether obvious or subtle, compromise the efficacy of algorithms in real-world applications.
At ImmuneWatch we have no doubt that accurate TCR–epitope binding prediction tools can bring immense potential benefits for the development of T-cell based diagnostics and therapeutics, which will ultimately benefit the patient. Rigorous validation of prediction scores and exclusion of negative data bias is an important part of bringing these models to reality.
The pitfalls of negative data bias for the T-cell epitope specificity challenge
Nature Machine Learning Intelligence
https://doi.org/10.1038/s42256-023-00727-0
Stay up to date
Subscribe to our newsletter to receive useful tips and information.
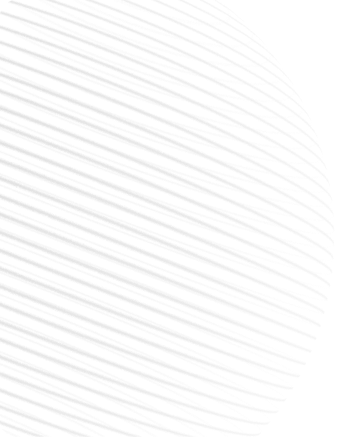